We’re exploring the frontiers of AGI, prioritizing readiness, proactive risk assessment, and collaboration with the wider AI community. Artificial general intelligence (AGI), AI that’s at least as capable as humans at most cognitive tasks, could be here within the coming years. Integrated with agentic capabilities, AGI could supercharge AI to understand, reason, plan, and execute…
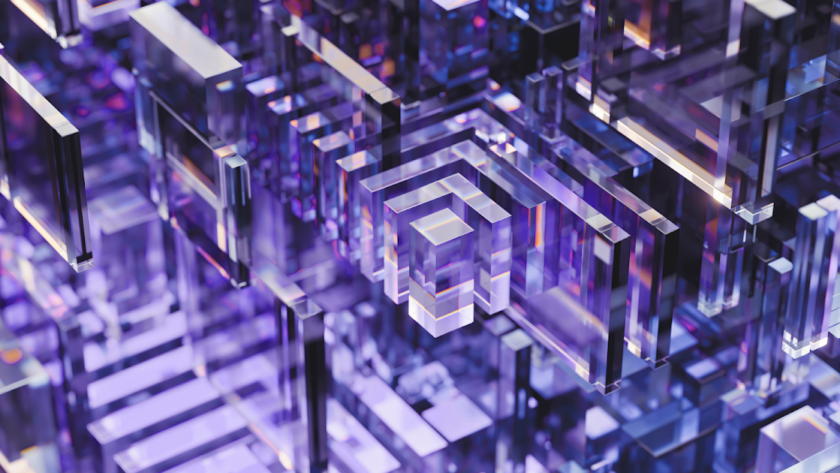